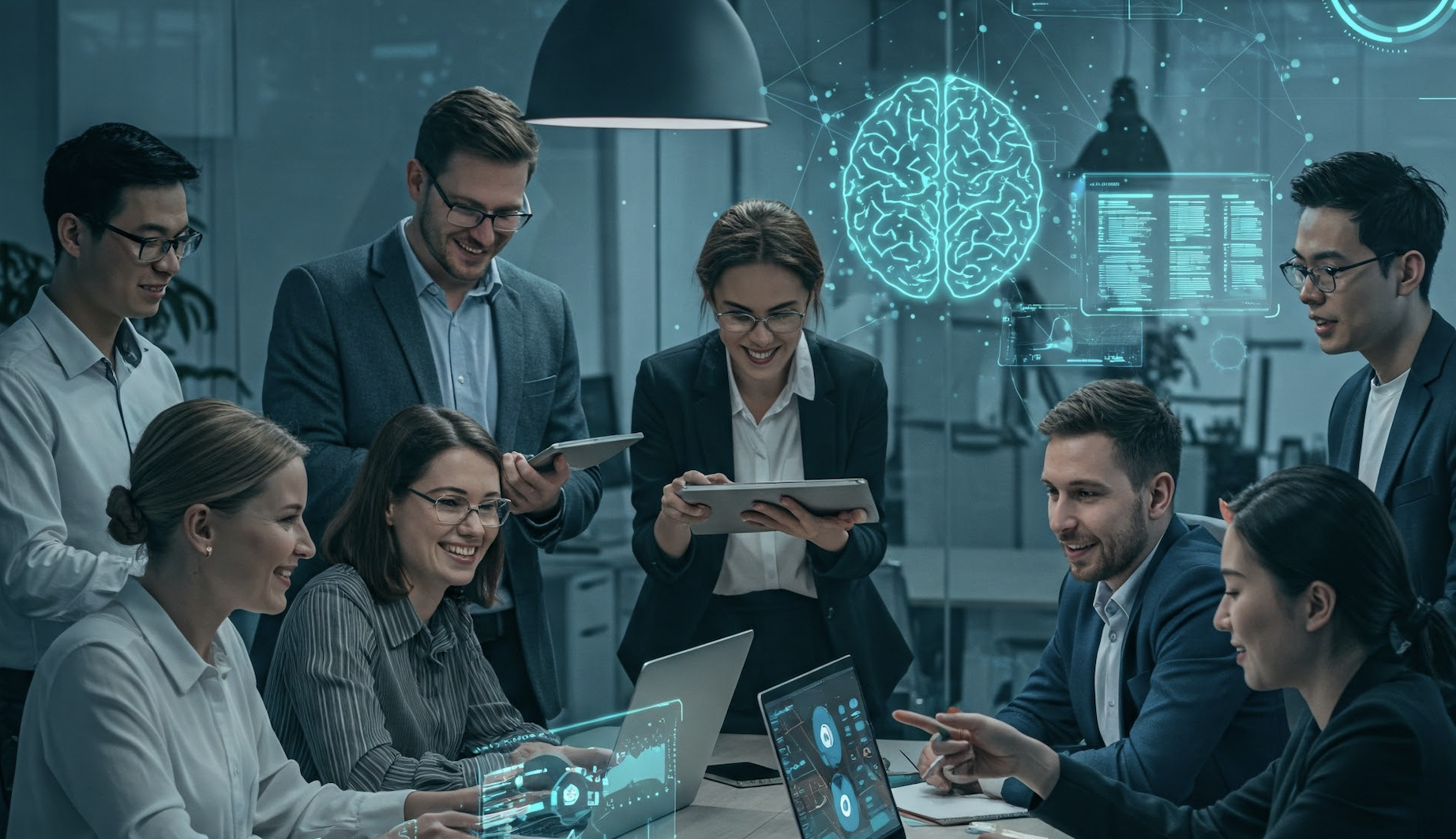
Introduction: Why AI Adoption at the Team Level Matters Now
The business world reached a turning point in early 2025. While large enterprises have been investing in AI for years, a new trend has emerged that's particularly relevant for organizations with 25-100 employees: team-level AI adoption.
According to recent data from Gartner's Enterprise AI Readiness Report, teams that have successfully implemented AI tools are experiencing productivity gains of 27-35% compared to peers still in the planning stages. This represents a significant opportunity for organizations to compete effectively against larger competitors.
The timing is perfect for three reasons:
1. More accessible technology: AI tools have become specialized for specific team functions and don't require massive IT resources
2. Decreasing costs: Implementation costs have dropped by nearly 50% since 2023, according to Forrester Research
3. Growing talent pool: Basic AI literacy is becoming common, making it easier to find and develop skilled team members
This guide is designed for leaders who want practical, actionable strategies for implementing AI at the team level—where the actual work happens and where the most immediate value can be realized.
The 2025 Reality: AI Adoption for Your Organization
What's Changed for Small-Medium-Sized Companies?
If you've been watching the AI space with interest but hesitation, 2025 marks a significant shift in what's possible for your organization:
Budget and control have shifted downward. In 2023, AI initiatives required centralized IT departments and specialized expertise. Today, according to MIT Technology Review's Q1 2025 Global AI Index, 58% of organizations report having distributed AI budgets, with individual departments and teams empowered to select and implement tools specific to their functions.
Implementation timelines have compressed dramatically. Organizations your size are now seeing implementation cycles of 6-8 weeks for team-level tools compared to the 6-8 months previously required. This means you can see results within a quarter rather than waiting half a year or more.
The tools have evolved to meet your needs. The market now includes thousands of specialized tools designed for specific team functions that don't require data scientists or AI engineers to implement. According to G2's 2025 AI Software Market Overview, 64% of today's team-level AI tools require minimal technical expertise, and 78% offer straightforward integration with common workplace systems.
The Productivity Gap Is Real and Growing
The difference between teams using AI effectively and those still relying entirely on human effort has become too significant to ignore:
• Teams using AI tools spend 37% less time on routine administrative tasks
• They access information and knowledge 42% faster
• Their forecasting and planning accuracy has improved by 29%
• They experience 31% fewer avoidable errors
These efficiency gains directly impact the bottom line and competitive position. A team of 10 people gaining even 25% more productivity effectively adds 2.5 people worth of output without increasing headcount.
These improvements vary by department type, with customer service teams seeing the highest impact (39% average productivity increase), followed by marketing (36%), operations (31%), and finance (27%), according to the Harvard Business Review Workplace Efficiency Index published in March 2025.
Why Now Is the Time for Your Teams to Adopt AI
The Business Case for Your Organization
The business case for team-level AI is compelling and immediate:
Do more with your existing team. Rather than hiring additional staff in a tight labor market, AI tools allow your current team members to significantly increase their output. Organizations implementing AI writing tools have increased content production by an average of 58% without adding headcount.
Make better decisions with limited information. Without the extensive data resources of larger competitors, AI tools help level the playing field by extracting more insights from the data you do have. AI analytics tools can identify patterns and opportunities in customer interaction data that previously required dedicated data science teams.
Respond faster in competitive situations. In competitive industries, speed often determines success. AI-enabled teams can generate proposals, respond to customer inquiries, and analyze market changes significantly faster than manual processes allow. According to McKinsey's State of AI 2025 report, AI implementation reduces response times by an average of 63% for common business processes.
Reduce costs in key operational areas. Operational efficiency directly impacts profitability. Teams using AI for process optimization, scheduling, and resource allocation are identifying cost-saving opportunities that were previously invisible. Deloitte's Digital Transformation Survey found that 58% of organizations using AI in operations reported identifying previously hidden efficiency improvements.
First-Mover Advantages
Implementing AI at the team level now creates several sustainable advantages:
Skill development lead time: Teams currently implementing AI are building expertise that typically takes 9-12 months to fully develop. By the time competitors begin their implementations, your teams will have already refined their processes and moved to more advanced applications.
Process optimization momentum: Early AI implementations typically identify 30-40% efficiency gains, but teams that have been using these tools for over a year report additional 15-20% gains in year two as they refine their processes and applications, according to BCG's 2025 AI Value Creation Report.
Talent attraction and retention: As AI skills become increasingly valuable in the job market, teams with established AI implementations are becoming talent magnets. The Society for Human Resource Management's 2025 Professional Development Survey found that 73% of knowledge workers prefer joining organizations with advanced AI tools over those still using primarily manual processes.
When Team AI Makes the Most Sense for Your Organization
Not all teams will see the same level of immediate impact. Based on implementation data, team AI adoption is most beneficial when:
• The team handles high volumes of structured data – Teams processing customer records, transaction data, support tickets, or content assets typically see the fastest ROI
• Repetitive decisions are common – Teams making frequent decisions using similar criteria can quickly benefit from AI-assisted decision support
• The team has defined processes – Teams with established workflows can more easily identify AI integration points
• Response speed impacts results – Teams where faster response creates competitive advantage benefit significantly from AI implementation
Prioritizing implementation for teams meeting these criteria ensures the quickest return on investment and builds momentum for broader adoption.
Assessing Your Team's AI Readiness
Before jumping into implementation, assess your team's readiness across five key dimensions. This will help you identify strengths to leverage and gaps to address before proceeding.

Technical Infrastructure: The Foundation
You don't need enterprise-level infrastructure, but certain basics must be in place:
- Cloud access and permissions: Most team AI tools are cloud-based. Ensure your teams have appropriate access rights and that your company security policies allow for these tools.
- Current software with API capabilities: Modern AI tools connect with existing systems via APIs. Evaluate whether your current tools offer accessible APIs, as this significantly impacts integration ease.
- Adequate internet connectivity: While obvious, this can be overlooked. AI tools typically require stable, reasonably fast internet connections for all team members.
- Basic endpoint devices: Most team AI tools run in browsers or lightweight applications. Standard business laptops or desktops are typically sufficient, though some graphics-intensive applications may require better hardware.
Data Readiness: Fuel for Your AI Engine
AI tools need data to provide value. Assess your situation realistically:
- Data availability: Identify whether your team has access to the data necessary for your priority use cases. Most successful implementations need at least a few months of historical data for reference.
- Data quality: Evaluate the accuracy and consistency of your team's data. Common issues include inconsistent formatting, duplicate records, and outdated information.
- Data permissions: With privacy regulations tightening, ensure your team has proper permission to use data for your intended purposes. This is particularly important for customer and employee data.
Skill Readiness: Preparing Your People
You don't need AI experts, but certain baseline capabilities help:
- Basic digital fluency: Team members should be comfortable with standard business software and willing to learn new tools. If your team struggles with existing technology, address those issues before adding AI.
- Learning orientation: Teams that value skill development adapt more quickly to AI tools. Assess whether your team regularly seeks out new knowledge and skills.
- Problem-solving mindset: The ability to troubleshoot issues and adapt to new workflows predicts implementation success. Teams comfortable with finding workarounds and solutions implement AI more effectively.
- At least 1-2 tech-savvy team members: Most successful implementations include at least a couple of team members with above-average technical aptitude who can support their colleagues.
Process Readiness: Creating the Context for Success
Your existing processes significantly impact implementation success:
- Documented workflows: Teams with clearly documented processes can more easily identify AI integration points. If your key processes exist only in people's heads, document them before implementation.
- Measurement mechanisms: Having baseline metrics for current performance allows you to measure AI impact accurately. Establish KPIs for key processes before implementation.
- Decision criteria clarity: AI excels at applying consistent criteria. If your team's decision processes are highly subjective or inconsistent, AI integration becomes more challenging.
Cultural Readiness: The Human Element
Team culture predicts implementation success more reliably than any technical factor:
- Openness to new approaches: Teams that regularly embrace new methods adapt to AI more quickly than those resistant to change.
- Psychological safety: Team members need to feel safe expressing concerns and asking questions. If people fear looking incompetent, they'll resist using new tools.
- Improvement mindset: Teams constantly seeking better ways to work adopt AI more successfully than those satisfied with the status quo.
- Leadership support: When team leaders actively champion new tools and demonstrate their use, adoption rates increase dramatically.
The 5-Phase Implementation Approach
Phase 1: Discovery and Use Case Definition
Start with business problems, not technology. This phase identifies where AI can create the most immediate value for your team.
What to do:
1. Conduct a task audit with your team to identify repetitive, time-consuming activities
2. Prioritize potential use cases based on:
• Time currently spent on the task
• Frequency of the task
• Impact on team results
• Implementation complexity
3. Define specific success metrics for each priority use case
4. Identify potential concerns or resistance points
Expected timeline: 1-2 weeks
Key deliverable: Prioritized use case document with specific success metrics
Phase 2: Tool Selection and Preparation
With clear use cases defined, you can evaluate specific AI tools based on your actual needs rather than getting distracted by flashy features.
What to do:
1. Define specific requirements based on your priority use cases
2. Research tools specifically designed for your team function and use case
3. Prioritize established tools with:
• Demonstrated results for organizations your size
• Straightforward integration with your existing systems
• Reasonable pricing models (typically $20-100 per user monthly for team tools)
• Solid security practices and compliance certifications
4. Request demonstrations focused on your specific use cases
5. Start with a single tool addressing your highest-priority use case
Expected timeline: 2-3 weeks
Key deliverable: Tool selection decision with implementation requirements
Starting with Enterprise Platforms You Already Use
Before exploring specialized AI tools, consider leveraging AI capabilities already available within your existing enterprise platforms. These offer an accessible starting point with minimal additional investment:
Microsoft 365 with Copilot:
If your organization already uses Microsoft Office applications, Copilot provides AI assistance across the entire suite. Key team-level benefits include:
• Content generation and summarization in Word and PowerPoint
• Data analysis and pattern identification in Excel
• Meeting transcription and action item extraction in Teams
• Email drafting and prioritization in Outlook
• Document discovery and insights across SharePoint
According to Microsoft's implementation data, teams using Copilot save an average of 7.4 hours per week through automated routine tasks, allowing team members to focus on higher-value work.
Google Workspace with Gemini:
For organizations using Google's productivity suite, Gemini offers AI capabilities throughout:
• Smart document creation and editing in Docs
• Formula generation and data analysis in Sheets
• Presentation design and enhancement in Slides
• Email composition and response suggestions in Gmail
• Meeting facilitation and summarization in Meet
Implementation data from Google indicates that Gemini users report 29% faster document creation and 32% more effective collaboration.
These enterprise platforms offer several advantages as your first AI implementation:
• Minimal additional training since teams already know the base applications
• Lower initial investment compared to specialized tools
• Broad applicability across multiple team functions
• Established security and compliance frameworks
• Foundation of AI experience that prepares teams for more specialized tools
Consider a "hybrid approach" by starting with these enterprise AI features for immediate productivity gains, then adding specialized tools for high-value, function-specific use cases as your team's AI comfort grows.
Phase 3: Team Preparation and Training
Even the best AI tools fail without proper team preparation. This phase ensures your team is ready for successful adoption.
What to do:
1. Identify 1-2 "AI champions" within your team who will receive advanced training
2. Develop a simple training approach with:
• Initial overview session (30-60 minutes)
• Hands-on practice with real work examples
• Quick reference guides for common tasks
• Regular check-ins during the first few weeks
3. Address concerns openly and honestly:
• Emphasize how AI will eliminate tedious tasks, not replace jobs
• Set realistic expectations about capabilities and limitations
• Encourage questions and feedback throughout the process
4. Create a simple change management plan:
• Communicate the "why" behind the implementation
• Establish clear timelines for rollout
• Define what success looks like
Expected timeline: 1-2 weeks
Key deliverable: Training plan and change management approach
Phase 4: Pilot Implementation
Start with a controlled implementation to validate your approach before rolling out to the entire team.
What to do:
1. Select 3-5 team members for the initial pilot
2. Focus on a single, well-defined use case
3. Set a specific timeframe (typically 2-3 weeks)
4. Provide enhanced support during this period:
• Daily check-ins during the first week
• Immediate troubleshooting of issues
• Regular feedback collection
5. Measure results against your defined success metrics
6. Identify and address process adjustments needed
Expected timeline: 2-3 weeks
Key deliverable: Pilot results and adjustments for full implementation
Phase 5: Full Implementation and Optimization
With lessons from the pilot, roll out to the full team and establish mechanisms for continuous improvement.
What to do:
1. Train the full team using insights from the pilot
2. Implement in phases if your team is larger (10+ people)
3. Establish regular check-ins during the first month
4. Create a feedback mechanism for ongoing improvement
5. Measure and communicate results using your defined metrics
6. Celebrate early wins and success stories
7. Begin identifying additional use cases based on initial success
Expected timeline: 2-4 weeks initial rollout, then ongoing optimization
Key deliverable: Full team implementation with continuous improvement process
High-Impact AI Applications for Different Team Types
While every organization is unique, certain AI applications have proven particularly valuable for teams. These examples can spark ideas for your own implementation.
Marketing Teams: Doing More with Limited Resources
For marketing teams typically stretched across multiple functions, AI tools provide leverage in key areas:
Content creation and optimization:
• AI-assisted writing for routine materials (product descriptions, email templates, social posts)
• Content performance prediction to focus efforts on high-potential topics
• Automated A/B test analysis to optimize messaging
• SEO optimization suggestions for existing content
Customer insights and targeting:
• Customer segmentation based on behavior patterns
• Personalization recommendations across channels
• Campaign performance prediction and optimization
• Competitor content analysis to identify gaps and opportunities
Implementation considerations:
• Start with content creation for faster, visible results
• Maintain human review for brand voice consistency
• Use AI suggestions as starting points, not final outputs
• Track time savings and content performance metrics
Sales Teams: Closing More Deals with Better Intelligence
Sales teams often lack the support resources of larger organizations. AI helps level the playing field:
Lead prioritization and management:
• Prospect qualification based on likelihood to convert
• Next-best-action recommendations for each prospect
• Follow-up timing optimization
• Account health monitoring and alert systems
Sales enablement and support:
• Customized proposal generation based on prospect needs
• Competitive intelligence gathering and analysis
• Objection handling suggestions based on prospect signals
• Meeting preparation briefings and talking points
Implementation considerations:
• Ensure CRM data is reasonably clean before implementation
• Start with a single high-impact application rather than complete transformation
• Provide clear guidelines on when human judgment should override AI suggestions
• Measure both efficiency metrics and sales outcomes
Customer Service Teams: Better Experience with Faster Resolution
Customer service teams often struggle with handling volume while maintaining quality. AI provides powerful support:
Ticket management and routing:
• Automatic categorization of incoming requests
• Priority scoring based on urgency and sentiment
• Suggested responses for common questions
• Similar case identification for faster resolution
Knowledge management and team support:
• Automated identification of knowledge base gaps
• Agent guidance during complex interactions
• Quality assurance and feedback on interactions
• Customer sentiment analysis and trend identification
Implementation considerations:
• Start with behind-the-scenes tools before customer-facing applications
• Build in clear escalation paths for complex issues
• Use AI to augment rather than replace human agents
• Focus on reducing agent cognitive load and stress
Operations Teams: Optimizing Process and Performance
Operations teams often manage complex processes with limited resources. AI offers significant efficiency gains:
Process optimization and monitoring:
• Bottleneck identification in workflows
• Anomaly detection for quality issues
• Resource allocation optimization
• Predictive maintenance and issue prevention
Planning and forecasting:
• Demand prediction based on multiple factors
• Scenario planning and simulation
• Inventory optimization recommendations
• Staffing and capacity planning
Implementation considerations:
• Start with monitoring applications before automated decision-making
• Validate AI recommendations against expert judgment initially
• Create clear processes for handling AI-identified exceptions
• Focus on proactive capabilities rather than just efficiency
Measuring Success: Proving the Value
Effective measurement is critical for guiding implementation and demonstrating value. Practical measurement approaches focus on business impact rather than technical metrics.
Focus on Business Outcomes, Not Tech Metrics
The most meaningful measurements connect directly to business performance:
Time recaptured:
• Hours saved on routine tasks
• Faster completion of key deliverables
• Reduction in overtime or weekend work
• Capacity created for higher-value activities
Quality improvements:
• Error reduction in key processes
• Consistency enhancements across team output
• Compliance improvement with standards
• Customer-reported quality perception
Business impact:
• Revenue increases from improved capabilities
• Cost savings from efficiency gains
• Customer satisfaction improvements
• Employee satisfaction and retention enhancement
Creating a Simple Measurement System
Measurement should be straightforward and actionable:
1. Establish clear baselines before implementation:
• Time studies for key processes
• Quality metrics for important outputs
• Team capacity and utilization measures
• Customer feedback and satisfaction scores
2. Define success metrics specific to your priority use cases:
• Select 3-5 key indicators per implementation
• Include both leading indicators (adoption, usage) and lagging indicators (business results)
• Ensure metrics are easily trackable without complex systems
3. Create a simple dashboard for tracking progress:
• Update at least monthly during initial implementation
• Include trend data to show progress over time
• Share with the team to maintain momentum
• Use results to identify additional optimization opportunities
Common Pitfalls and How to Avoid Them
Organizations implementing team AI typically encounter predictable challenges. Awareness helps you navigate these obstacles successfully.
Starting with Technology Instead of Problems
Many teams fall into the trap of beginning with exciting AI capabilities rather than specific business challenges.
Warning signs:
• Conversations focus on AI features rather than business outcomes
• Difficulty explaining how the AI tool will change specific workflows
• More excitement about the technology than the problems it solves
How to avoid it:
• Always begin with the specific challenge being addressed
• Evaluate AI tools exclusively against defined business requirements
• Create clear before/after comparisons for each workflow
• Assign business metric owners for each implementation objective
Inadequate Training and Support
Insufficient preparation is a primary cause of implementation failure, particularly for organizations with limited training resources.
Warning signs:
• Team members expressing confusion about when or how to use the tools
• Inconsistent usage patterns across similar roles
• Workarounds developing to avoid using the new tools
• Declining usage trends after initial adoption
How to avoid it:
• Create role-specific quick-start guides focused on daily applications
• Establish peer support mechanisms for ongoing assistance
• Schedule brief reinforcement training 2-3 weeks after initial implementation
• Develop a simple internal knowledge base for common questions
• Ensure leaders visibly use and advocate for the tools
Unrealistic Expectations About AI Capabilities
Misunderstanding what current AI can and cannot do leads to disappointment and abandonment.
Warning signs:
• Frustration with AI limitations that should have been anticipated
• Disappointment with accuracy despite normal performance
• Over-reliance on AI for judgment calls requiring human context
• Resistance developing after minor errors despite overall value
How to avoid it:
• Clearly communicate current AI limitations from the beginning
• Provide examples of typical errors and how to handle them
• Establish when human judgment should override AI suggestions
• Implement progressively to build confidence
• Celebrate incremental improvements rather than expecting perfection
Getting Started: Your First 30 Days
Week 1: Assessment and Planning
• Complete team AI readiness assessment across all dimensions
• Conduct task audit to identify potential high-value use cases
• Select 1-2 priority use cases with clear business impact
• Establish baseline metrics for current performance
Weeks 2-3: Selection and Preparation
• Research tools specifically designed for your use case
• Evaluate 2-3 options against your specific requirements
• Select and purchase initial tool (start with single-team implementation)
• Identify and begin developing 1-2 AI champions within your team
• Develop simple training approach and change management plan
Week 4: Initial Implementation
• Conduct team training sessions focusing on practical applications
• Launch controlled pilot with subset of team members
• Establish daily check-ins and feedback mechanisms
• Address initial technical and workflow challenges
• Capture early wins and success stories
Beyond 30 Days: Expansion and Optimization
• Expand to full team based on pilot learnings
• Measure impact against baseline metrics
• Refine workflows and processes based on team feedback
• Establish regular review and optimization rhythm
• Begin planning for additional use cases based on initial success
Conclusion: The Competitive Advantage
The window of opportunity for team-level AI adoption in 2025 represents a critical inflection point for organizations. While large enterprises have dominated the AI conversation for years, the real competitive advantage now lies in practical, team-level implementation that delivers immediate value.
This shift in the AI landscape creates a rare opportunity to compete effectively against larger organizations by:
Achieving enterprise-level productivity with your existing teams. The efficiency gains from effective AI implementation allow your teams to produce at levels previously requiring significantly larger headcounts.
Moving faster than larger competitors. Your ability to implement quickly and adapt rapidly provides an agility advantage over enterprises with more complex approval and integration requirements.
Creating sustainable capability advantages. Teams that successfully implement AI develop institutional knowledge and refined processes that create compounding benefits over time.
Attracting and retaining top talent. As AI skills become increasingly valuable, providing team members with cutting-edge tools becomes a significant recruitment and retention advantage.
The frameworks presented in this guide provide a straightforward approach to capturing these advantages without requiring enterprise-level resources or specialized expertise. By focusing on business problems rather than technologies, preparing your team thoroughly, and measuring results systematically, you can achieve the productivity and capability gains that will position your company for success.
The time to begin your team's AI journey is now. Start with the readiness assessment, identify your high-value use cases, and take the first steps toward implementation. The most successful organizations of 2025 and beyond will be those that embrace these technologies not as futuristic possibilities, but as practical tools for today's challenges.
Ready to take the next step? Start with our Team AI Readiness Assessment to identify your strengths and improvement opportunities.