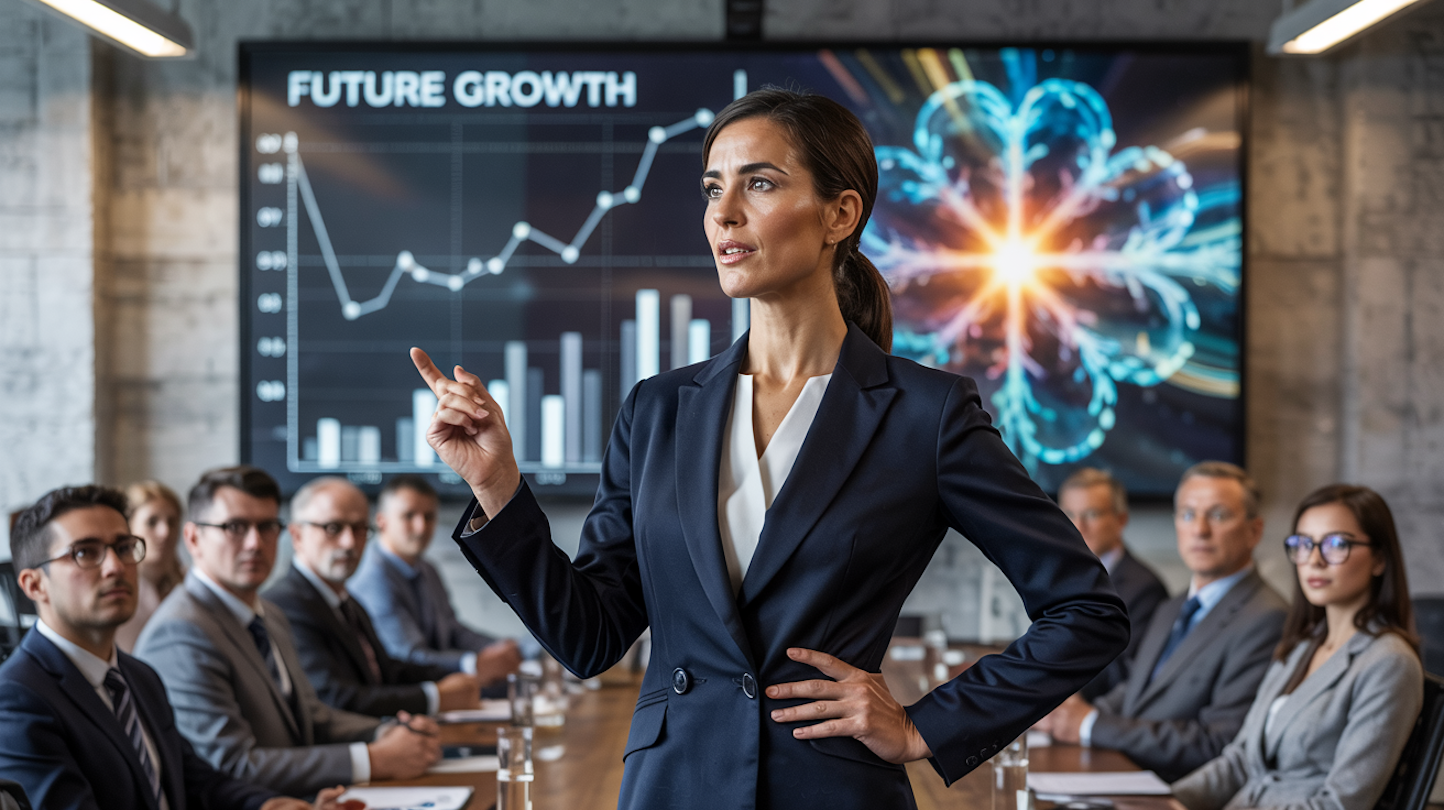
Introduction: The ROI Paradox
Picture this scenario: A forward-thinking CEO recognizes AI's transformative potential for her organization. She's read the reports, attended the conferences, and understands that AI will fundamentally reshape her industry. Yet when she proposes significant AI investments to her board, she faces the inevitable question: "What's the ROI?"
This moment—where visionary understanding meets practical fiscal responsibility—creates a tension familiar to leaders across industries. On one hand, traditional ROI calculations seem inadequate for capturing the full value of technologies that promise to transform entire business models. On the other hand, organizations cannot responsibly allocate resources without some measure of expected returns.
I encounter this tension regularly in my work with executives implementing AI. Many find themselves caught between two seemingly contradictory positions: the AI evangelists claiming "this will change everything" and the financial stewards asking for clear, quantifiable returns. Both perspectives have validity, yet neither alone provides adequate guidance.
This tension is not a dichotomy we must accept, but rather a paradox we can resolve. The path forward isn't choosing between "immeasurable transformation" and "narrow ROI calculations," but developing a more sophisticated measurement framework that satisfies both visionary and practical concerns.
Human-centered AI implementation—an approach that prioritizes enhancing rather than replacing human capabilities—isn't just philosophically sound. When properly measured, it proves financially prudent as well. The organizations seeing the greatest returns from AI are precisely those measuring its impact most intelligently.
In this article, I'll introduce an expanded framework for measuring AI's value across four dimensions: operational value, capability value, human capital value, and strategic positioning value. This approach bridges philosophical AI transformation with practical business metrics, providing leaders with a more comprehensive understanding of AI's true impact.
My goal isn't to dismiss the ROI question, but to elevate it—to help executives ask better questions that lead to better decisions about one of the most consequential technologies of our time.
Part 1: Why Traditional ROI Calculations Fall Short for AI
The Historical Pattern of Technological Disruption
We've been here before. Every transformative technology in business history has challenged conventional ROI thinking, creating winners and losers based largely on how organizations approached value measurement.
Consider the early commercial internet. Companies that evaluated websites purely on direct sales metrics in 1998 dramatically underinvested compared to those who recognized the broader transformation of customer relationships. Amazon lost money for years while traditional retailers showed positive ROI from their modest online investments—until the accumulated capability gap became insurmountable.
Similarly, organizations that evaluated early mobile technology solely on cost savings missed the customer experience revolution that fundamentally reshaped industries. Banks that measured mobile banking through traditional branch-focused metrics fell years behind competitors who understood the broader transformation underway.
AI represents a similar inflection point, but with even greater potential for measurement myopia. The pattern is clear: traditional financial metrics, while necessary, have consistently proven insufficient for evaluating technologies that transform how value is created.
The Three Blind Spots of Traditional AI ROI Calculations
Blind Spot #1: Capability Development Value
Standard ROI calculations struggle to capture how AI builds organizational capabilities that don't immediately appear on balance sheets. These capabilities—the ability to process more information, make better decisions, and adapt more quickly—often create the greatest long-term value.
Consider how AI implementations create learning effects and compound knowledge gains. Unlike traditional technology investments where value depreciates over time, AI systems frequently become more valuable as they accumulate data and organizational learning.
One manufacturing client initially justified their predictive maintenance AI solely on reduced downtime metrics. Two years later, the accumulated insights into equipment performance had transformed their product design process—a value stream entirely missed in the original ROI calculation but worth far more than the initial maintenance savings.
This capability development creates exponential rather than linear returns, yet traditional ROI models inherently use linear projections based on known use cases at the time of investment.
Blind Spot #2: Human Capital Impacts
AI significantly affects talent attraction, retention, and engagement—factors traditionally considered HR concerns rather than technology ROI components.
Research consistently shows that knowledge workers increasingly prefer opportunities with organizations using advanced AI tools in their field. According to Salesforce's 2023 State of IT report, 71% of IT leaders say their employees are more likely to stay at their company as a result of adopting automation and AI tools, and 85% of IT leaders report that employees are actually requesting more AI solutions for their daily work.
Beyond retention, AI transforms the nature of work itself. When implemented with a human-centered approach, AI can shift routine tasks to more strategic, creative work—creating substantial value through reduced turnover and higher productivity.
Blind Spot #3: Opportunity Costs and Competitive Positioning
Perhaps the most significant blind spot in traditional ROI thinking involves opportunity costs and competitive positioning. The gap between AI leaders and laggards is widening across industries, creating a competitive divide that becomes increasingly expensive to bridge.
Organizations waiting for "proven ROI" before investing substantially in AI face a mathematical challenge: by the time the returns are unequivocally proven in their industry, competitors have established significant leads in data, talent, and capabilities.
In rapidly changing industries, companies that implemented AI before clear ROI evidence existed now operate with substantial advantages in market responsiveness and customer experience. These advantages become increasingly difficult for competitors to overcome as capabilities compound over time.
This creates a fundamental paradox in traditional ROI thinking: by the time the evidence satisfies conventional return thresholds, the strategic opportunity has often diminished or disappeared entirely.
Part 2: The Expanded Measurement Framework
Beyond Efficiency: Four Dimensions of AI Value
To address these blind spots, leaders need an expanded framework that captures AI's multidimensional impact. This framework measures value across four dimensions, providing a more comprehensive understanding of returns.
Dimension 1: Operational Value
This dimension encompasses traditional metrics that financial stakeholders immediately recognize: cost reduction, time savings, error reduction, and throughput increases. While insufficient alone, these metrics remain essential.
Effective measurement in this dimension includes:
• Process automation savings: Direct labor cost reduction from automated tasks
• Throughput improvements: Increased capacity without additional resources
• Quality enhancements: Reduced error rates and rework requirements
• Resource optimization: More effective allocation of existing resources
The key to measuring operational value effectively isn't abandoning these metrics but contextualizing them within a broader framework. Organizations should capture immediate efficiency gains while acknowledging they represent only one value component.
Implementation approaches that don't require perfect precision include:
• Sampling methodologies that measure representative processes rather than comprehensive tracking
• Threshold measurements that confirm minimum ROI requirements while avoiding analytical paralysis
• Comparative measurements against industry benchmarks when absolute precision proves elusive
One healthcare provider effectively measured operational value by tracking time savings across representative processes, finding significant efficiency improvements without attempting to monetize every minute saved across the organization.
Dimension 2: Capability Value
This dimension measures how AI enhances organizational learning, adaptation, and knowledge creation—capabilities that drive future value creation beyond current processes.
Effective capability value metrics include:
• Skill development velocity: How quickly team members acquire AI-related expertise
• Innovation cycle acceleration: Reduced time from idea to implementation
• Decision quality improvements: Better outcomes from enhanced information processing
• Knowledge accessibility: Improved ability to leverage organizational expertise
• Adaptability indicators: Enhanced capability to respond to market changes
Professional services firms have begun measuring capability value by tracking decision quality across client engagements, finding that teams with AI support consistently deliver recommendations with fewer revisions and higher client-reported implementation success.
The key to effective capability measurement is establishing clear baselines before implementation and tracking developments through both quantitative and qualitative methods, including expertise assessments, decision quality reviews, and innovation metrics.
Dimension 3: Human Capital Value
This dimension captures AI's impact on the people who ultimately drive organizational success: employees, partners, and talent networks.
Effective human capital metrics include:
• Work quality transformation: Shifts from routine to strategic activities
• Employee experience improvements: Satisfaction with tools and workflows
• Talent attraction and retention impacts: Reduced turnover and improved recruiting
• Professional development acceleration: Enhanced skill acquisition and career advancement
• Collaboration and innovation indicators: Increased cross-functional work and idea generation
Forward-thinking organizations track human capital value by measuring the percentage of employee time spent on creative problem-solving versus routine tasks, with AI implementation often increasing strategic work while maintaining or improving overall output.
Measuring this dimension effectively requires close collaboration between technology and HR functions, combining traditional engagement metrics with specific technology impact assessments.
Dimension 4: Strategic Positioning Value
This final dimension measures how AI affects an organization's competitive position and future options—often the most significant long-term value driver.
Strategic positioning metrics include:
• Market perception changes: Customer and partner awareness of enhanced capabilities
• Competitive differentiation indicators: Unique capabilities enabled by AI
• Future option creation: New business possibilities enabled by AI capabilities
• Adaptability to market changes: Enhanced ability to pivot and respond
• Innovation capacity: Organizational ability to develop new offerings
Organizations at the forefront of AI implementation are finding that significant portions of their current revenue derive from offerings that didn't exist before their AI capabilities were developed—a value stream entirely invisible to traditional ROI calculations.
Creating a Balanced AI Value Scorecard
To implement this expanded framework, organizations should develop a balanced scorecard that:
- Weights these four dimensions based on organizational context and strategic priorities
- Sets appropriate timeframes for different types of returns, recognizing that value accrues at different rates across dimensions
- Communicates the expanded framework effectively to stakeholders accustomed to traditional ROI
The weighting of dimensions should reflect your organizational reality. A fast-moving consumer goods company might emphasize operational and strategic positioning dimensions, while a professional services firm might place greater emphasis on human capital and capability dimensions.
Timeframes should similarly reflect organizational context while acknowledging natural development sequences. Operational value typically emerges first (0-6 months), followed by capability value (3-12 months), human capital value (6-18 months), and strategic positioning value (12+ months).
The key is creating a comprehensive view that neither overpromises immediate returns nor understates long-term strategic impact.
Part 3: Implementing Human-Centered AI with Measurable Returns
Starting with Measurable Pilots
The expanded measurement framework doesn't imply abandoning fiscal discipline. Rather, it suggests a more sophisticated approach to implementation that balances immediate returns with long-term value creation.
Effective AI implementation begins with carefully selected pilot initiatives that:
• Demonstrate operational value within 3-6 months
• Build capabilities that enable subsequent initiatives
• Affect processes visible to key stakeholders
• Provide clear before/after comparison opportunities
The measurement framework should be established before implementation begins, with clear baseline metrics across all four value dimensions. This approach prevents the common problem of scrambling to demonstrate value after the fact with inadequate "before" data.
Retail organizations have effectively balanced quick wins with strategic development by implementing inventory optimization AI that delivered immediate operational savings while building data capabilities that enabled subsequent customer experience initiatives—creating a value stream that justified continued investment.
The Phased Measurement Approach
Measuring AI's full impact requires a phased approach that tracks different value dimensions as they emerge:
Phase 1: Efficiency metrics (0-3 months)
• Focus: Operational value dimension
• Key metrics: Process time reduction, cost savings, quality improvements
• Measurement approach: Direct comparison to baseline operations
• Purpose: Demonstrate basic functionality and immediate returns
Phase 2: Capability metrics (3-9 months)
• Focus: Capability value dimension with continued operational tracking
• Key metrics: Decision quality improvements, knowledge accessibility, process adaptability
• Measurement approach: Capability assessments, knowledge utilization tracking
• Purpose: Demonstrate developing organizational capabilities
Phase 3: Human capital metrics (6-12 months)
• Focus: Human capital dimension with ongoing operational and capability tracking
• Key metrics: Work quality transformation, employee experience impacts, talent indicators
• Measurement approach: Surveys, work sample analysis, turnover and recruitment tracking • Purpose: Demonstrate people-level impact and organizational benefits
Phase 4: Strategic positioning metrics (12+ months)
• Focus: All four dimensions with emphasis on strategic positioning
• Key metrics: Market perception, competitive differentiation, future options
• Measurement approach: Market analysis, strategic capability assessment
• Purpose: Demonstrate sustainable competitive advantage
This phased approach maintains stakeholder confidence through early operational wins while building the case for more substantial, strategic value over time.
Practical Measurement Tools and Techniques
Implementing the expanded framework requires practical measurement approaches that balance rigor with feasibility:
Baseline establishment methodologies
• Process timing studies for operational baselines
• Capability maturity assessments for capability baselines
• Employee experience surveys for human capital baselines
• Competitive positioning analysis for strategic baselines
Mixed-method approaches
• Quantitative metrics for operational and capability dimensions
• Qualitative assessments for human capital and strategic dimensions
• Case studies that illustrate impact across multiple dimensions
• Executive interviews that capture perceived value from key stakeholders
Executive dashboards
• Balanced presentation of all four value dimensions
• Progressive disclosure from high-level indicators to detailed metrics
• Trend visualization showing development over time
• Comparison against initial projections and industry benchmarks
Continuous feedback mechanisms
• Regular user feedback on AI tools and integration
• Process owner assessments of impact and opportunities
• Customer and partner perception tracking
• Cross-functional impact assessment
Leading healthcare organizations have implemented this measurement approach by creating quarterly AI value assessments that combine operational metrics (time savings, error reduction), capability metrics (decision support utilization, knowledge access improvements), human capital metrics (physician and staff satisfaction, recruitment effectiveness), and strategic positioning metrics (patient perception, service differentiation).
Part 4: Reframing the Executive Conversation
From "What's the ROI?" to Better Questions
The expanded measurement framework enables more sophisticated executive conversations about AI investments. Rather than focusing narrowly on traditional ROI, leaders can ask better questions that lead to better decisions:
"How will this AI initiative affect our organizational capabilities?"
"What impact do we expect on our talent strategy and employee experience?" "How might this change our competitive positioning over the next 2-3 years?"
"What new options or opportunities might this create beyond the immediate use case?"
"How will we measure success across different timeframes and value dimensions?"
These questions guide the conversation toward multidimensional value without abandoning financial discipline. They create space for both visionary thinking and practical measurement.
Leading technology companies have transformed their AI governance by replacing traditional "ROI threshold" approval processes with balanced assessments that require initiatives to demonstrate value in at least three of the four dimensions, with operational value mandatory for most projects but not necessarily the primary justification.
Making the Case to Different Stakeholders
The expanded framework also allows for tailored communication with different executive stakeholders:
The CFO conversation
• Lead with operational value metrics that speak to financial priorities
• Connect capability development to future cost avoidance and revenue potential
• Demonstrate how human capital improvements affect financial fundamentals
• Frame strategic positioning in terms of market share and pricing power
• Use industry benchmarks to contextualize investment levels
The CHRO conversation
• Emphasize human capital dimension and workforce transformation
• Connect AI implementation to talent strategy and employee experience
• Demonstrate how capability development affects career progression
• Show how operational improvements create capacity for higher-value work
• Align measurement with existing human capital development frameworks
The CEO conversation
• Focus on balanced value across all four dimensions
• Emphasize strategic positioning and competitive differentiation
• Connect AI capabilities to core strategic priorities and challenges
• Demonstrate how implementation builds organizational adaptability
• Present clear milestones and governance frameworks
Effective stakeholder engagement requires translating the expanded framework into language that resonates with each executive's priorities while maintaining a consistent overall approach.
Anticipating and Addressing Pushback
Even with an expanded framework, you may encounter resistance to this more sophisticated approach to AI value. Common objections include:
"This seems too complicated to implement."
Response: Start with basic measurements in each dimension rather than comprehensive tracking. Begin with qualitative assessments in dimensions that prove challenging to quantify. Implement in phases, starting with operational metrics and progressively adding dimensions
"Our board only cares about hard numbers."
Response: Include traditional financial metrics while progressively introducing additional dimensions. Use case studies and competitive examples to illustrate the risk of narrow measurement. Provide parallel reporting with both traditional and expanded frameworks during transition
"We don't have bandwidth for complex measurement."
Response: Focus on high-impact metrics rather than comprehensive coverage. Leverage existing data collection where possible rather than creating new processes. Implement measurement proportional to investment size and strategic importance
The key is balancing measurement sophistication with practical implementation constraints, recognizing that even an imperfect expanded framework provides better guidance than traditional ROI alone.
Frequently Asked Questions About AI Value Measurement
"How do we measure returns when AI's impact spans multiple departments?"
This common challenge reflects AI's tendency to create value that crosses traditional organizational boundaries. Effective approaches include:
Cross-functional measurement teams
Establish measurement governance that includes representatives from all affected departments, with shared metrics that track end-to-end value rather than departmental contributions alone.
Value flow mapping
Create visual representations of how AI-enabled value flows across departmental boundaries, identifying both origin points and ultimate impacts regardless of organizational structure.
Organizational vs. departmental frameworks
Implement dual measurement systems—organizational metrics that capture total value and departmental metrics that track local impact—while recognizing that the sum of departmental benefits often understates total organizational value.
For example, a customer service AI might reduce call handling time (customer service department metric) while simultaneously improving product design through feedback analysis (product development department metric) and increasing customer lifetime value (overall organizational metric).
The key is avoiding measurement approaches that create artificial boundaries around value that naturally flows across the organization.
"What timeframe should we use for calculating AI ROI?"
AI initiatives typically deliver value across multiple timeframes, making single-period ROI calculations problematic. More effective approaches include:
Staged ROI expectations
Establish different return thresholds for different time horizons: immediate operational returns (0-6 months), mid-term capability returns (6-18 months), and long-term strategic returns (18+ months).
Rolling measurement windows
Implement measurement approaches that continually update as new value emerges rather than fixing assessment at a predetermined endpoint.
Milestone-based rather than time-based frameworks
Define success based on capability development milestones rather than calendar dates, recognizing that some value emerges on capability achievement rather than predetermined schedules.
Organizations that rigidly apply quarterly or annual ROI expectations to transformative technologies typically undershoot value by focusing on immediate returns while missing compounding long-term benefits.
Healthcare organizations have found that initial AI ROI calculations based on 12-month returns often significantly underestimate actual returns revealed by later retrospective analysis, as capability development and strategic positioning benefits emerge beyond the original timeframe.
"How do we account for AI's impact on intangible assets?"
Intangible assets—including brand value, intellectual property, organizational knowledge, and customer relationships—often represent the most significant value created by AI implementations. Effective measurement approaches include:
Proxy metrics for intangible value
Identify measurable indicators that correlate with intangible asset development, such as customer retention rates (for customer relationship value) or employee knowledge assessment scores (for organizational knowledge value).
Scenario valuation techniques
Develop scenario-based valuations that estimate how enhanced capabilities affect future options and opportunities, even when direct measurement proves challenging.
Competitive benchmarking
Use competitive comparison to assess relative capability development and its potential market value, particularly when absolute measurement is difficult.
Market perception analysis
Track how customers, partners, analysts, and other stakeholders perceive capability enhancements, using sentiment analysis and direct feedback.
Professional services firms have begun measuring knowledge asset development by tracking how AI affects time-to-competence for new professionals, finding that AI-enabled knowledge systems can significantly reduce the development cycle—a value they translate into both cost savings and revenue potential.
"What if our competitors are using traditional ROI and gaining board approval more easily?"
This question reflects a legitimate concern: might organizations with more sophisticated measurement be disadvantaged in securing investment approval? Effective responses include:
Competitive intelligence approaches
Develop a nuanced understanding of competitor investment approaches beyond their public statements, recognizing that many organizations publicly emphasize traditional ROI while privately employing more sophisticated frameworks.
Education on competitive risk
Help stakeholders understand how narrowly focused ROI requirements may create approval "wins" but implementation "losses" as competitors with more nuanced approaches secure sufficient investment for transformative capabilities.
Hybrid approval frameworks
Implement approval processes that satisfy traditional ROI requirements for initial funding while establishing expanded measurement for ongoing investment, creating a bridge between immediate fiscal responsibility and long-term value creation.
The key is helping stakeholders understand that the goal isn't maximizing the number of approved AI projects but maximizing long-term value creation, which may require more sophisticated measurement approaches than competitors employ.
"How much precision do we need in our AI value measurements?"
Leaders often struggle with the appropriate level of measurement precision, particularly when AI initiatives affect complex systems with many variables. Effective approaches include:
The false precision problem
Educate stakeholders about the dangers of false precision, where exact-looking numbers create illusions of certainty in inherently uncertain domains. In many cases, directional accuracy proves more valuable than decimal-point precision.
Confidence levels and ranges
Replace point estimates with confidence ranges that honestly reflect certainty levels, such as "70% confident of 15-25% improvement" rather than promising exactly "20% improvement."
Precision proportional to materiality
Apply measurement precision proportional to investment size and strategic importance, with larger and more consequential initiatives warranting more sophisticated measurement.
Organizations that demand excessive precision for AI value often create analytical paralysis that delays implementation while competitors move ahead with directionally correct but less precisely measured initiatives.
"How do we balance human-centered values with shareholder expectations?"
Leaders sometimes perceive tension between human-centered approaches to AI and shareholder value maximization. In reality, evidence increasingly supports their alignment:
The growing alignment with shareholder value
Share research demonstrating that human-centered AI implementations consistently deliver superior financial returns through enhanced adoption, more effective implementation, and greater workforce benefits.
Environmental, Social, and Governance (ESG) considerations
Connect human-centered AI approaches to broader ESG commitments, noting the growing importance of these factors to institutional investors and their alignment with effective AI governance.
Long-term vs. short-term framing
Position human-centered approaches as long-term value optimization rather than short-term profit reduction, emphasizing how enhancing human capability builds sustainable competitive advantage.
Narrative strategies for stakeholders
Develop communication approaches that explicitly connect human-centered values to business outcomes, helping stakeholders understand how these approaches drive financial performance rather than constraining it.
Research is increasingly showing that human-centered AI implementation isn't just ethically sound but financially prudent, with companies emphasizing human-centered AI governance often outperforming their peers in multiple performance metrics.
Conclusion: Leading with Intelligence, Measuring with Wisdom
The Balanced Perspective
Throughout this article, I've argued that traditional ROI calculations, while necessary, prove insufficient for measuring AI's full impact. The expanded framework—encompassing operational value, capability value, human capital value, and strategic positioning value—provides a more comprehensive understanding of how AI transforms organizations.
This isn't about abandoning measurement discipline but enhancing it. Organizations that measure AI's impact effectively make better investment decisions, implement more successfully, and create more sustainable competitive advantage than those relying solely on traditional ROI metrics.
The goal isn't replacing financial stewardship with unaccountable technological evangelism, but rather elevating our approach to measurement in ways that match the transformative potential of the technology itself.
The Human-Centered Advantage
Organizations implementing AI with human capability at the center consistently outperform those focused solely on automation and efficiency. This advantage stems from several sources:
• More effective adoption and implementation through better alignment with human workflows
• Enhanced capability development as AI augments rather than replaces human judgment
• Stronger talent attraction and retention through meaningful work preservation
• More sustainable competitive advantage through capabilities that are harder to replicate
These benefits create a flywheel effect where initial advantages compound over time. Human-centered implementation generates better data, builds stronger capabilities, attracts better talent, and creates more options—advantages that accelerate with each implementation cycle.
This approach directly addresses the measurement blind spots that plague traditional ROI thinking, capturing the full spectrum of value creation rather than just the immediately quantifiable components.
Practical Next Steps
Leaders can begin implementing this expanded measurement approach immediately through several concrete actions:
- Audit current AI measurement approaches Review how your organization currently evaluates AI investments and implementations, identifying blind spots in current value assessment.
- Identify one pilot initiative for expanded measurement. Select an ongoing or planned AI initiative to apply the expanded framework, establishing baselines across all four value dimensions.
- Create a stakeholder education plan. Develop materials that help key stakeholders understand the limitations of traditional ROI and the benefits of the expanded approach.
- Implement phased measurement rollout. Begin with operational metrics familiar to stakeholders while progressively introducing additional dimensions as the organization builds measurement capabilities.
- Establish regular review cadence. Create a structured process for reviewing value creation across all dimensions, adjusting implementation based on emerging insights.
These steps allow organizations to evolve their measurement approach while maintaining stakeholder confidence and implementation momentum.
The Future-Ready Organization
As AI continues to transform business, the gap between sophisticated and simplistic measurement approaches will widen. Organizations that measure effectively will make better investment decisions, implement more successfully, and capture more value than those constrained by traditional ROI thinking.
The future belongs not just to organizations that adopt AI, but to those that understand its multidimensional impact and measure it accordingly. As leaders, our challenge is matching our measurement sophistication to the technology's transformative potential—leading with intelligence and measuring with wisdom.
The ROI question isn't wrong, just incomplete. By expanding our understanding of AI's value creation, we make better decisions today that position our organizations for success tomorrow.